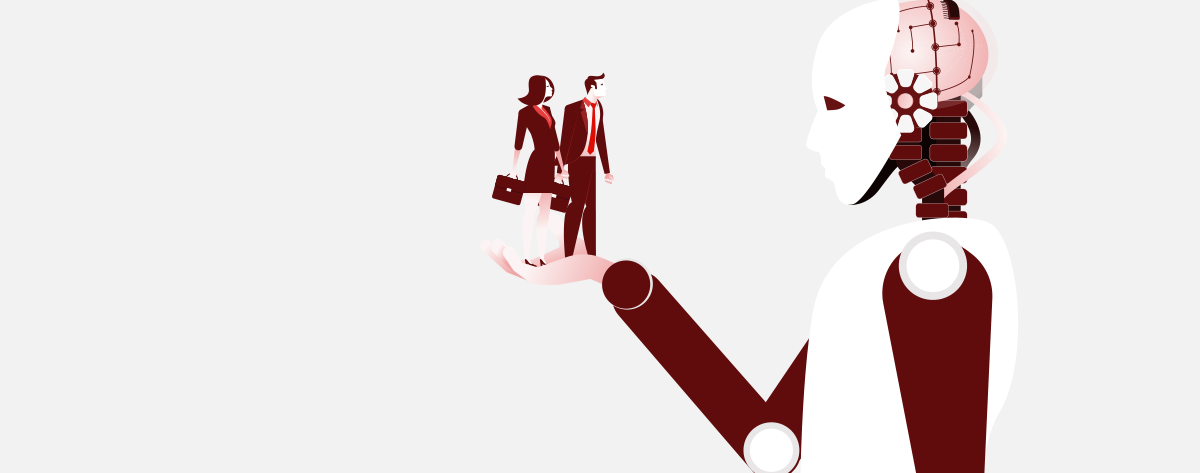
Harnessing The Evolution Of Artificial Intelligence In The Fight Against Gender Bias
Technology’s lack of human error is its sustained edge over human-driven decisions. But when it comes to gender parity, is that really so?
Biases are a form of human error too. And when 50% of the world’s population suffers from this bias, it’s more than an error. Technology can do a lot in designing an equitable future for men and women. Provided, of course, that it is not built on these biases. Reports show how spurious, gender-biased data is being fed into artificial intelligence models.
A strong example of this predisposition lies in Natural Language Processing (NLP) models. These models are the foundation of Apple’s Siri and Amazon’s Alexa. Their neutrality and impartiality is questionable, to say the least. Word-embeddings pre-disposition the data to bias, by assigning stereotypical gender roles. As HBR puts it, “Doctor for male and nurse for female.”
According to Dr. Daphne Koller, cofounder Coursera and adjunct CS faculty member at Stanford University, “You do a search for C.E.O. on Google Images, and up come 50 images of white males and one image of C.E.O. Barbie. That’s one aspect of bias.”
There is even a dearth of representative talent. Only 12% of machine-learning innovators and researchers are women. Computer vision systems used for gender recognition also reflect gender biases.
Another source of gender bias is only subjecting data to a first-level analysis. This means ignoring ‘red flag’ questions in the ‘how and why’ of data.
Understanding Gender Bias in Artificial Intelligence
Biases already exist in the way technology professionals design and deploy AI solutions. Gender recognition systems report higher error rates in recognizing women than men. These error rates vary with demographics and other characteristics. The worst-hit casualties are women of color, doubling the impact of both race and gender.
Even if this is unconscious bias on the solution designer’s part, AI should have a way of reversing it.
Reasons for Bias in Artificial Intelligence
Artificial intelligence solutions, as we see above, have a gender bias. Answering ‘why’ and ‘how’ will help reverse it.
The first issue is the incidence of error itself. Many variables can explain fluctuating error counts. The first is data paucity. Along with being insufficient, representative datasets are also imbalanced.
Three more factors that add gender bias to AI models are:
1) Skewed, Inadequate Datasets
Datasets are often designed with a few nominated demographic classes. So even the training datasets are not generalizable. This makes it difficult for models to scale data for excluded demographic classes.
Machine learning can fight gender bias if training models use less homogenous datasets.
2) Manmade Training Labels
AI models can train data. How they do this, though, still depends on human input, vis-a-vis machine learning.
How do we fix this?
Data scientists must subject data labels to cognitive fallacy tests. Before assigning them to datasets. If done after, the biases will get encoded into the core of the machine learning model.
Data architects must ensure data estimation is safe from misclassification and assumptions.
3) Machine-Learning Models Characteristics
Completely numeric datasets are somewhat easier to manage for gender bias. It gets even more complex with unstructured datasets. Or data that relies on other data forms, such as speech recognition.
Scientists formerly used the analytics of “taller speakers” to program speech models. Taller people generally tend to have lower-pitched voices. i.e. The models could ‘better’ detect male voices sharing similar characteristics.
Why are there are more errors in recognizing the higher-pitched voices of females? This is why.
How To Avoid Gender Bias in Machine-Learning Models
Although the output of biased data is unfair, its motive is not. It is usually the result of skewed quantification. The solution, then, is to have diversity at the core of dataset development, not as an add-on.
Over here, data scientists need to take caution against over-fitting their datasets. Diversity for the sake of diversity does as much harm as not having diversity at all.
Also, take note that exception labeling helps identify the actual issue of representation. Even within affirmative data models, women at risk are under-represented. Thus, gender equality comes under the risk of disguising the privilege of some women. These women do not represent the whole.
Artificial Intelligence, The Great Equalizer
Thus, continual replenishing and widening the foundation of datasets helps weaken gender bias. Another way is to treat model training as a truly reiterative exercise. For instance, predictive modeling can forecast increased female representation. (In, say the consumption of financial products, like loans). Perhaps it could start by asking a different question: What was the ratio of men vs women who were denied loans? That’s how AI’s real powers can be used in making workplaces (and economies), more inclusive.
References
1. https://www.nytimes.com/2019/11/19/technology/artificial-intelligence-bias.html
2. https://www.internationalwomensday.com/Missions/14458/Gender-and-AI-Addressing-bias-in-artificial-intelligence
3. https://edition.cnn.com/2019/11/21/tech/ai-gender-recognition-problem/index.html
4. https://hbr.org/2019/11/4-ways-to-address-gender-bias-in-ai
5. https://www.catalyst.org/research/trend-brief-gender-bias-in-ai/
6. https://www.sciencedaily.com/releases/2019/07/190710121649.htm
7. https://www.reuters.com/article/us-amazon-com-jobs-automation-insight/amazon-scraps-secret-ai-recruiting-tool-that-showed-bias-against-women-idUSKCN1MK08G